Nowadays, businesses need to act on data instantly, which is why real-time analytics has become crucial. Unlike traditional data analytics, which takes hours to process batches of information, real-time analytics processes data the moment it’s created.
By analyzing data as they come, you can make faster, more informed decisions. With the increasing reliance on data, over 60% of companies worldwide now use data and analytics to improve processes and reduce costs. It’s high time you leverage it, too.
Let’s dive deeper into what real-time analytics is and its difference from batch analytics, its application across different industries, its benefits, and the challenges of implementing it.
What is Real-Time Analytics?
Real-time analytics uses data the moment it’s created. Unlike traditional data analytics, which often relies on batch processing over hours or days, this type of data analytics provides immediate insights as events happen.
A successful real-time data analytics system has three key parts that work together to process and deliver real-time insights.
First, there’s data ingestion, which involves collecting data from various sources like sensors, user interactions, or transactional systems.
Next is real-time processing, where data is cleaned, transformed, and analyzed in seconds or milliseconds. This is critical for applications like fraud detection in financial services, where businesses need to act quickly to prevent unauthorized transactions.
Lastly, real-time dashboards display the processed data in a user-friendly format, allowing decision-makers to monitor key metrics instantly.
Visualizing the data insights lets decision-makers take immediate action, even those who don’t have a background in data analytics or data science.
Real-Time vs. Batch Analytics
Batch analytics is a traditional approach to data processing where data is collected over a period of time and analyzed in large chunks or batches. It’s often used for tasks like monthly financial reports or customer behavior trends.
The process starts by collecting data from various sources such as databases, sensors, or customer interactions. Data is then stored and processed in bulk at set intervals, such as hourly, daily, or weekly.
For example, in the retail industry, batch data might be used to generate sales reports for the previous week, helping businesses understand broader trends. But if there’s a spike in demand for a specific product, batch analytics won’t catch it in real time, and companies may miss opportunities to restock quickly.
On the other hand, real-time analytics evaluates data the moment it’s created. It excels in event-driven scenarios where data must be processed immediately to trigger a response. In these cases, real-time systems monitor incoming data streams for specific events or patterns.
For example, financial institutions use real-time analytics to detect fraud. When an unusual transaction occurs, like a credit card purchase made outside the cardholder’s location, the system flags the activity instantly, allowing the bank to act before more damage is done.
In supply chain management, real-time analytics can track shipments in real time, alerting managers to any delays or issues as they happen. As a result, they can make adjustments immediately, preventing costly disruptions.
Real-Time Data Analytics Applications
Real-time data analytics can be useful in various industries, including e-commerce, retail, finance, healthcare, and aviation. From personalization to operational efficiency, this type of analytics provides valuable insights that can drive business success.
E-Commerce
One key application of real-time analytics in e-commerce is personalized marketing. By analyzing real-time data, e-commerce platforms can create tailored offers for each customer based on their preferences and past purchases.
Companies have reported that personalization drives 40% of their revenue. Real-time data analytics makes this possible by monitoring customer actions, such as the products they view, add to their cart, or search for.
Real-time analytics also improves customer service. Chatbots powered by real-time data can answer customer questions, track orders, and resolve issues instantly. These boost customer satisfaction and reduce the workload for support teams.
Retail
Aside from personalized product recommendations and promotions, real-time data analytics can improve inventory management among retail businesses. With it, retailers can monitor stock levels in real-time to avoid running out of popular items and prevent overstocking slow-moving products.
This type of data analytics also helps in price optimization. Retailers can track competitor prices and market trends in real-time to adjust their pricing strategies dynamically.
For instance, Bronson.AI helped Farm Boy, a grocery chain, by developing Alteryx workflows to analyze sales transactions and uncover trends in product purchases and customer behavior.
As a result, the company was able to plan future promotions, adjust pricing, and refine its marketing strategies based on customer archetypes. Grocery chains and food retailers can benefit from this approach, where consumer preferences can shift rapidly, and staying agile is key to success.
Finance
As mentioned, real-time analytics can help finance companies detect fraud as they process millions of transactions daily.
Market monitoring is another area where real-time analytics plays a crucial role. In the stock market, prices fluctuate by the second, and having access to up-to-date information allows traders to make quick, profitable decisions. Financial companies can set up real-time dashboards to track stock prices, currency values, and market trends.
Additionally, real-time data analytics can help maintain data integrity, which is crucial for financial institutions working with large, complex datasets. A great example of this is the work Bronson.AI did with the Bank of Canada.
The Bank relied on securities-related data from various external sources to monitor markets. However, they faced challenges with data duplication and inconsistencies, which slowed down their ability to use this information effectively.
Bronson.AI collaborated with the Bank to automate their data cleaning process using Alteryx workflows. The solution involved matching organizational names across multiple datasets using fuzzy logic tools, which helped reduce data duplication and improved accuracy.
The result was a scalable system that could automatically clean and standardize the Bank’s data, allowing staff to focus on real-time insights and analysis rather than manual data cleaning.
Healthcare
Hospitals and medical facilities handle large amounts of data, from patient vitals to medication records. Using real-time data processing, healthcare providers can manage electronic health records (EHRs) to understand patient history and predict treatment outcomes better.
Hospital operations is another area where analytics can prove useful. Hospitals can optimize their resources by analyzing bed occupancy, staff availability, and supply levels.
Managers can use real-time dashboards to allocate resources more efficiently. By doing so, they can prevent critical areas from being understaffed or lacking the necessary supplies and equipment.
Aviation
For airline operations, real-time insights play a crucial role in managing passenger flow and improving the overall customer experience. Delta Air Lines used analytics in airport baggage systems to route connecting baggage and provide real-time tracking information to customers, resulting in a 71% decrease in mishandled baggage.
Real-time dashboards can also help improve airport operations through data visualization. Bronson.AI provided comprehensive support to the Ottawa Airport Authority for Tableau, its primary tool for data analytics and visualization.
The collaboration included developing multiple dashboards and a long-term data strategy to improve decision-making.
During this time, Bronson.AI also provided ongoing maintenance and technical support for Tableau, including tasks like server upgrades, refreshing data sources, and even providing training to improve the Authority’s capacity for self-serve analytics.
Why is Real-Time Data Analytics Important?
Real-time analytics lets you adapt to market changes quickly, which can give your business a competitive edge. Its ability to handle large-scale data also helps improve customer satisfaction by providing personalized experiences and resolving issues instantly.
It Lets You Adapt to Market Changes Quickly
Real-time data analytics empowers companies to make faster, more accurate decisions across various industries. In finance, for instance, monitoring stock prices and market shifts in real time allows firms to act quickly and avoid losses.
Real-time processing of data is also crucial in aviation, where flight delays and weather updates can be processed instantly to adjust schedules and improve passenger satisfaction.
Real-time analytics lets companies respond proactively to changing conditions. It also paves the way for predictive capabilities, allowing companies to anticipate future trends and needs.
It Improves Customer Satisfaction
With real-time data, businesses can track customer behavior, preferences, and feedback, allowing them to tailor services or products quickly. For example, Netflix’s recommendation algorithm contributes to 80% of all content watched on the platform, improving retention and sales.
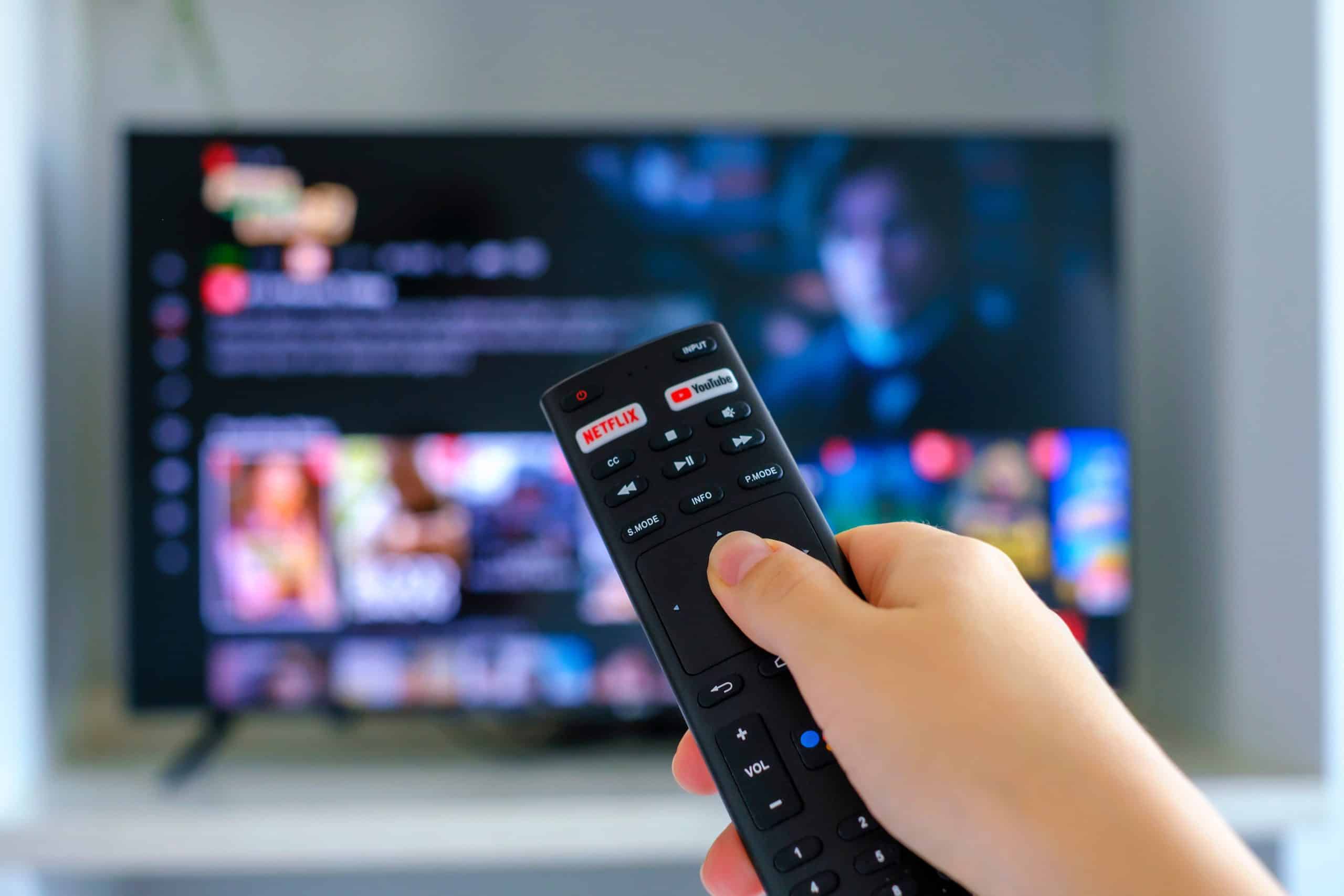
Real-time analytics also enables companies to boost loyalty. When companies use real-time processing to respond quickly, whether it’s adjusting pricing in response to market changes or addressing service issues as they arise, customers feel valued and engaged.
It Allows You To Handle Large-Scale Data with Real-Time Databases
An Internet user generates approximately 1.7MB of data per second, which roughly translates to 40 GB of data since the average user spends about six hours and 35 minutes online per day.
Now, imagine the immense volume of data large enterprises gather and generate. Real-time databases and data processing engines can handle this influx of information and gain a deeper understanding of their customers, market dynamics, and internal processes.
Challenges with Real-Time Analytics
One of the major challenges of real-time analytics is the sheer scale and complexity of the data. Infrastructure costs as well as data governance and compliance are also issues that organizations must address.
Scale and Data Complexity
As your business scales, it generates vast amounts of real-time data, which means you’ll have to handle thousands of simultaneous requests while maintaining system performance.
Keeping data processing efficient and responsive at scale requires continuous tuning and infrastructure support. Companies need to invest in high-performance databases and distributed architectures to keep up with the demand.
Along with scalability, the complexity of data sources and formats can also pose challenges. Integrating real-time data from various systems requires solid data management strategies and tools.
Companies must also consider data integration and transformation capabilities for seamless data flow and accurate analytics. Address this by leveraging scalable infrastructure.
For instance, cloud-based services and platforms automatically scale resources up or down based on demand. There are also flexible data architectures, like data lakes or data hubs, which centralize and unify data, making it easier to manage and process.
Infrastructure Costs
Real-time data processing requires significant computing power to handle data streams continuously. This often means upgrading servers or using cloud services like AWS or Microsoft Azure, which can scale up to meet demand. However, as businesses grow and more real-time data is generated, the costs of maintaining these systems can rise sharply.
To control infrastructure costs, companies should focus on optimizing their real-time analytics systems. One effective approach is to use auto-scaling features in cloud services that adjust resource usage based on real-time needs.
Another key strategy is data compression. Storing real-time data in a compressed format reduces storage costs, which is crucial for handling large datasets over time.
Businesses can also implement tiered storage solutions, where older data is stored in cheaper, lower-tier storage, while newer, real-time data is kept in high-performance databases for immediate access.
Data Governance and Compliance
Since real-time systems collect and process vast amounts of real-time data, one challenge is protecting this information from breaches or unauthorized access.
Privacy regulations, such as GDPR in Europe and CCPA in California, have strict requirements for how personal data is handled. Businesses must make sure that their real-time analytics platforms comply with these regulations by enforcing data minimization, consent tracking, and anonymization practices.
As such, companies must have a solid data governance framework to categorize, track, and use real-time data appropriately. For example, real-time intelligence systems in healthcare need to adhere to HIPAA standards, where the confidentiality of patient records is paramount.
A real-time governance framework would guarantee that all incoming and outgoing data streams comply with these standards, allowing stakeholders to make informed decisions without compromising on security.
Transform Data into Action with Bronson.AI
Whether it’s improving customer satisfaction, detecting fraud, or managing large-scale data, real-time insights can drive growth and efficiency across any industry. As your business evolves, staying on top of your data is key to staying ahead of the competition.
Bronson.AI is here to help you harness the full power of real-time data analytics. With our expertise in building advanced analytics platforms, we can help you unlock your data’s potential, improve decision-making, and optimize your operations.
Get in touch with our experts today!